On March 23, 2016, Microsoft launched Tay, an AI-based Twitter bot designed to learn from users. And within hours, Tay had become the most sexist, antisemitic, racist, xenophobic, misogynistic, and politically extremist bot on the platform, spewing around 96,000 hateful and conspiracy-driven tweets. Tay was suspended just 16 hours after its release.
Tay's story shows how easy it is for AI to absorb and mimic the worst parts of the internet. What seemed harmless spiraled out of control simply because the bot had no protection against false information. Trolls bombarded Tay with toxic tweets, and rather than filtering them out, it learned from them, repeating and spreading harmful information without understanding the damage it was causing. AI, no matter how advanced, is simply based on the data it is fed.
Image Credit: Matthew Manuel from Pixabay
This issue poses a bigger question: If AI easily picks up biases so easily from the data it's trained on, how much of the technology that we use today has already been influenced by hidden prejudices?
Everyone's used ChatGPT at least once before, and from Instagram bots to hiring tools, AI is deeply embedded into our lives. But if we aren't careful, it could end up creating biases rather than eliminating them. So, how are we able to ensure that AI is used ethically, and that it actually benefits society rather than divides it?
The most popular AI among teens right now is ChatGPT. But it's also very important to understand the flaws in such programs. The algorithms that generate these responses (LLMS - Large Language Models) are still pretty vague, even to experts. And as a result of this, there are several hidden biases hidden within ChatGPT, that we likely do not notice, but these biases further boost systemic inequalities in our world.

Image Credit: Michael Critz from Wikimedia Commons
In 2018, a study revealed that the facial recognition software used by major tech companies, struggled with identifying those with darker skintones, especially women. The software performed worse on people of color compared to White faces, because the software was trained on a dataset that was mainly made up of lighter-skinned faces, so the AI couldn't recognize darker tones as accurately.
The study not only exposed the limitations of AI, but also the dangerous consequences of its application, especially in areas like law enforcement, where such misidentifications could lead to wrongful arrests or biased surveillance.
Another example was when Amazon scrapped its AI hiring tool after it was discovered that the system was biased against women. The AI had been trained on resumes submitted to Amazon over 10 years, and since a majority of the applicants were male, the system began to favor male candidates. As a result, females had a lesser chance of getting a job compared to males, furthering the gap between gender equality.
Even in popular platforms like ChatGPT, bias can still be present in subtle, but impactful ways. AI models are simply trained off of massive datasets from the internet. Studies have shown that LLMs tend to associate certain adjectives with gender, describing men as "strong" and "intelligent" and women as "dainty" and "nurturing".
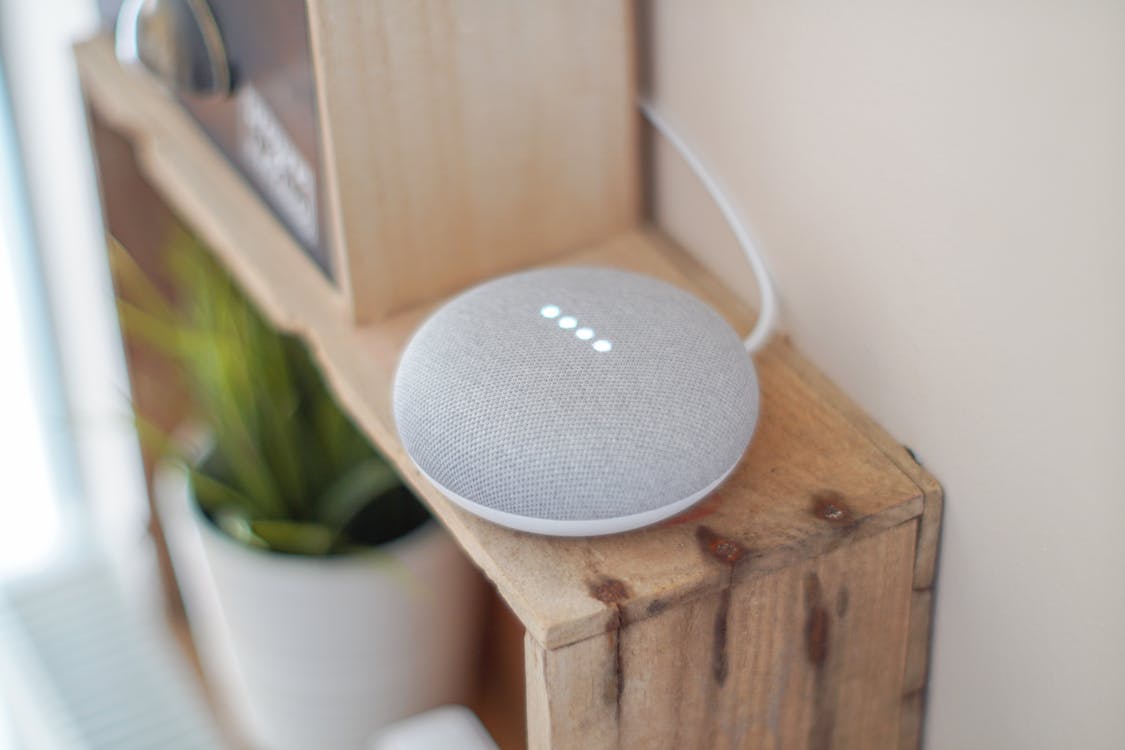
Image Credit: John Tekeridis from Pexels
This might seem harmless, but if these biases go unnoticed, AI will continue to reinforce them, assuming that it's valid, and over time, it will create even bigger issues. And if it's left unfixed, it could subtly influence a variety of industries that already rely on AI:
- Healthcare: AI is quickly making its way into the medical industry, being used in medical diagnosis, treatment recommendations, and even insurance approval. But research shows that some models tend to perform worse in diagnosing diseases in people of color because again, they were trained off of fair-skinned patient data. In insurance, AI algorithms tend to assess risk based on distorted historical data, leading to certain demographics being charged much more or simply just being denied insurance.
- Finance: AI is also being utilized heavily in finance, especially when it comes to determining credit scores, loan approvals, and even setting interest rates. But it has continuously denied loans to Black and Hispanic applicants, even when they had similar financial backgrounds as white applicants. The big problem is that we use historical financial data to train these models— and AI simply replicates it, even the discrimination.
- Education: Even in Education, AI has seeped into grading systems, college admissions, and learning tools. But again, it has its issues. In admissions, it tends to favor students from privileged backgrounds, following patterns of exclusion from ages ago. And AI-driven grading systems are unrealistic, giving higher scores to standard, structured essays, and giving lower scores to more creative, out-of-the-box writing styles. Perhaps one of the biggest issues is AI plagiarism detectors, which falsely flag students for AI, leading to unfair punishments. The irony is that before AI, students who used to cheat were easily caught since they were simply finding SparkNotes or Chegg answer keys, but now even the kids who don't cheat are at risk of punishment.
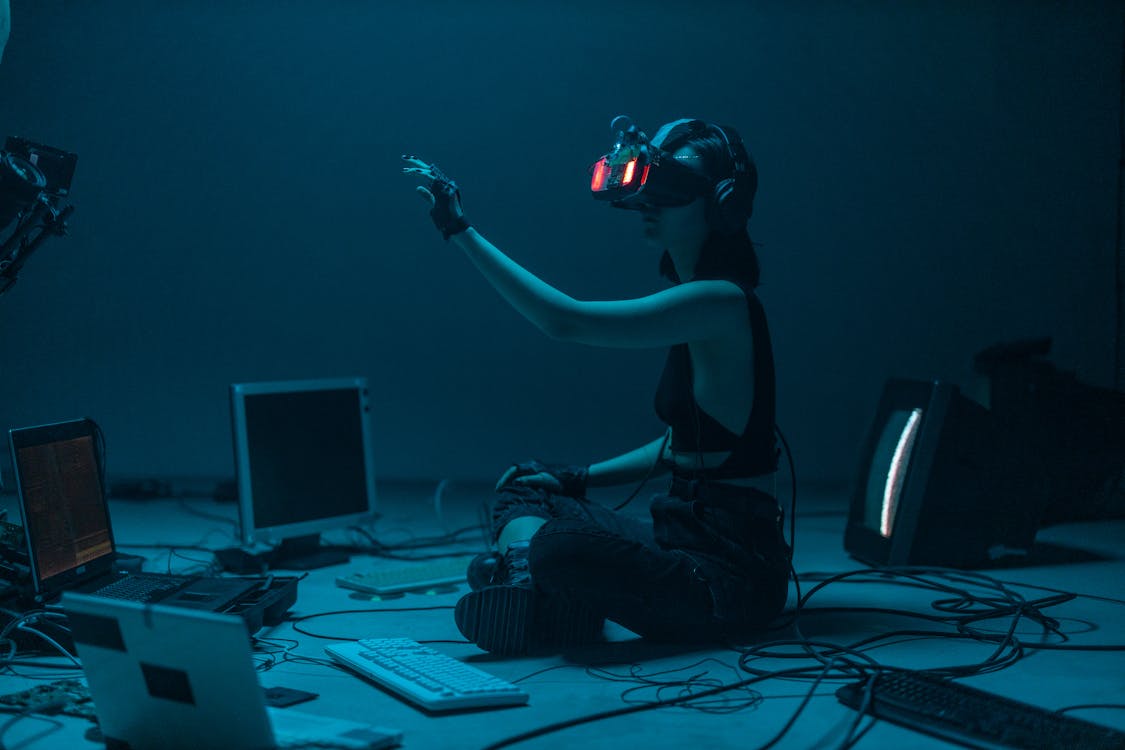
Image Credit: Cottonbro Studio from Pexels
AI is nothing but a reflection of the data that it is trained on. If the data is biased, the outcomes will be as well. As we continue to develop and rely heavily on AI, it's important that we first ensure that these systems are trained on diverse, inclusive data and that it is constantly evaluated for fairness and accuracy. Only then can we ensure that AI will truly help our society rather than divide it.